How to pair sensor technologies with the right extraction method
Properly combine NIR, AIR jet sorters, and robots to achieve sorting success
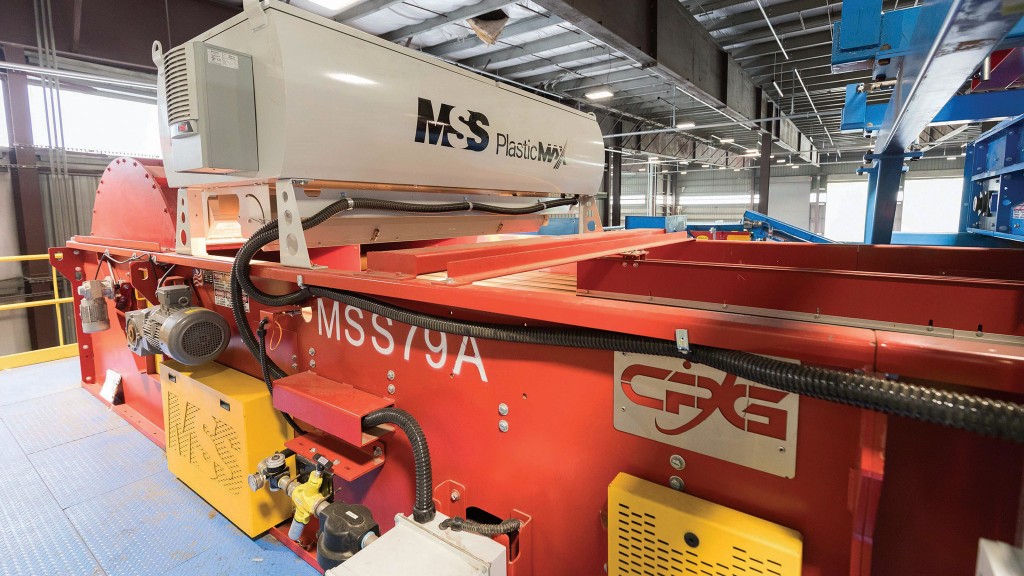
Recently, artificial intelligence (AI) and robotics have been getting a lot of attention. For some people, robotics and AI are synonymous. However, robotics is not AI, and AI is not robotics. AI seeks solutions to difficult problems related to human abilities, while robotics aims to automate physical, repetitive tasks. Additionally, robots are not the only mechanical technology that AI can be paired with. In some cases, the application dictates the need for more robust extraction, such as the air jets commonly used with optical sorters, which have proven their value in MRFs over decades.
At MSS, we are of the firm belief that sensing technology needs to be completely decoupled from extraction technology. One really has nothing to do with the other. A MRF operator needs to consider the best sensor technology to identify the targeted items in a mixed material stream, and then pair it with the most appropriate extraction method required to physically remove the targeted items.
Consider your sensor
The sensors used most commonly in mass sorting MRF applications are near-infrared (NIR), colour, and metal. Depending on the application, it could require just one or possibly a combination of these individual sensor technologies to achieve the sorting goal. The performance of these sensors can be enhanced by adding other sensor technologies, such as AI.
NIR and AI sensors can provide vast amounts of data and statistics to MRF operators for monitoring and operational improvement purposes. AI's deep-learning capability is complementary to NIR, and its deeper levels of available granularity provide finer classifications of individual product and material categories.
Furthermore, AI sensors build on the identification capabilities that NIR does not have, most prominently sorting items like cat food cans and cooking foil from used beverage cans (UBCs) and polyethylene terephthalate (PET) bottles from PET thermoforms. NIR sensors can truly identify material by chemistry, while AI sensors cannot.
AI sensors work like the human eye, deducing what an item might be based on what it looks like; it cannot determine if a bottle is made from PET or high-density polyethylene (HDPE), it only does so indirectly. AI sensors also cannot make the distinction between some PET and PP thermoformed containers or UBCs that have PET shrink sleeves, versus those with directly printed graphics. These are tasks that conventional NIR sensors, in combination with metal detectors, are well-suited to.
Conveyor and decision speeds are additional considerations. NIR sensors can go up to 1,000 feet per minute, while AI starts experiencing issues when the belt is moving faster than 300 feet per minute. Additionally, the decision speed, or the time between detection and extraction of an item, is about 10 times faster for NIR than AI sensors. As far as working width is concerned, AI sensors typically are used on up to 60-inch-wide belts. Therefore, if you want to go wider, you'll need to double the number of AI sensors. On the other hand, NIR sensors on optical sorters can be used on conveyor belts that are as wide as 112 inches.
Extraction options
The suction cups commonly used on robots are one form of extraction technology. The air jets used with optical sorters are another form. But the options don't end there; extraction technologies also can include clamps or diverters, for instance.
When determining the best extraction technology for a particular application, consider the effective picks per minute. We define effective picks as those where the effector successfully puts the correct target item into the dedicated chute, not just the number of movements or ejections. Based on the data we have available from all our installations, our air jet optical sorters can achieve over 1,000 effective picks per minute from a 112-inch-wide acceleration belt, whereas a robot might be able to achieve about 60 effective picks per minute (out of 90 possible movements), making it 15 times slower.
If the material targeted by a robot is nicely spread out on the conveyor belt, robots can perform well. However, if the targeted items are bunched up or their percentage in the input stream increases, robots are challenged to keep up. This leads to the pass stream being unnecessarily contaminated, or the recovery rate of the targeted items being much lower.
The geometry of the robotic arm's effector also is a factor. Common bellows-type suction cups work better with two-dimensional material, while three-dimensional items are difficult to pick up with these small suction cups. Air jets, on the other hand, can effectively eject 3D items.
When it comes to cost per pick, air jets have been shown to be about 10 times less expensive than suction cups. If we assume that 16,500 natural HDPE bottles comprise a ton, an MRF would spend $19 per ton sorted using a robot with a suction-cup effector. With air jets, that cost would be well under $2 per ton.
One area where robots can excel is their ability to sort multiple output fractions at once. While we normally recommend using single-eject configurations for most common MRF applications for air jet sorters, using a robotic arm allows three to four individual fractions to be sorted at the same time.
Application examples
On an aluminum quality control line where the goal is to sort non-UBC metals and non-metals from the UBCs, the number of required picks per minute exceeds any robot's capacity even in smaller MRFs. Therefore, the removal of the non-metals becomes a priority for the AI, which leaves the UBCs contaminated with non-UBC metal items.
The same occurs on PET QC lines where the goal is to sort thermoforms and non-PET from the PET bottles. With the percentage of thermoforms in the PET fraction rising, robots simply cannot keep up anymore. Combining AI sensor technology with air ejector extraction has proven more effective to achieve the required true picks per minute.
Other recent installations put AI-robot combinations on PE QC lines, where NIR-based conventional optical sorters are ejecting "all PE." Not only does PE include milk jugs and detergent bottles, but it also includes any PE-based tubs and lids. Because the AI does not know the difference between a PE or polypropylene (PP) lid, it's identifying and picking out perfectly acceptable PE lids because it thinks they are PP (based on the assumption that all lids are PP). In this scenario, not only does the robot itself potentially have to limit capabilities, but the AI is not the correct sensor for the application.
Furthermore, if the robot is deployed to also sort natural PE from colour PE at the same time, these incorrect picks take away the picks required to colour sort the PE. This leads to the much more valuable natural PE ending up in the colour PE, reducing the overall commodity value in the MRF.
An MRF operator should consider a technology platform that pairs the right type of sensor with the appropriate extraction method for the task at hand. The decision of whether AI or NIR should be used for a sorting function in a MRF needs to be carefully considered, as does the extraction method. While these technologies can be complementary, a robot cannot replace an optical sorter.
Felix Hottenstein is the sales director at MSS which develops and deploys automated sensor technologies for the waste management and recycling industry as part of CP Group.
Company info
300 Oceanside Drive
Nashville, TN
US, 37204
Website:
magsep.com
Phone number:
615-781-2669